The why
Mineral exploration involves a series of decisions about where to allocate resources towards making a discovery. At each decision point, exploration geoscientists, their managers and company board will want reliable information with which to make those decisions with confidence. Having a strong, data-rich geological rationale as the foundation for any decision will make the technical aspect of exploration an easier problem to solve.
With all the hype around artificial intelligence, it might be easy to look at new machine learning tools as either at best a gimmick with little real value, or at worse a threat to established, seemingly successful practices and processes.
In reality, AI is like every other tool: in skillful hands it can be used towards great purpose.
In the context of mineral exploration, we are now on the cusp of a revolution in which AI tools will forever change the way exploration is undertaken. New AI tools can assimilate, analyse and utilise vast amounts of geoscientific data. They can be asked to make predictions about aspects of a mineral system of interest such as the location of geological features such as alteration zones, or structural controls, or indeed the location of mineralisation itself.
A tool like this is extremely valuable.
Not because the AI will be able to replace geological thinking. Rather, AI can dispassionately provide data-driven hypotheses about the location and likelihood of geological features. Making decisions around testing those hypotheses is and will always remain the job of the geoscientist, who is able to contextualise the AI outputs within the real world framework of timeframes, land access, data reliability, and other variables that are impractical to account for in an AI system.
Out of the box thinking is often the step change that has led to many great discoveries. What if there was a way to fast track that type of thinking? Again, AI tools can provoke the type of deep thinking that can deliver previously unrecognised correlations, with the resulting insights that come with new ideas. For example, recently Fleet Space Technologies’ geoscientists were working with an AI prospectivity tool that determined a correlation between radiometric data and potential mineralisation in a situation where the mineralised rock masses were hundreds of meters below the surface. This unusual correspondence led the geoscientists to revisit the radiometrics data and develop an understanding that structures within the basement were being expressed as very subtle features in the radiometrics, potentially as neotectonic effects over older structures.
This is just one example.
In reality, when viewing multimodal data in which more than three dimensions are at play, the human mind finds it very difficult to establish connections across disparate data types. This is one of the great strengths of AI.
Moreover, we are all subject to psychological limitations. For example confirmation bias where we prefer an outcome that confirms our existing beliefs. Confirmation bias can be problematic, leading to missed opportunities. For example a bias derived from prevailing geological models that said gold would not be found in sedimentary rocks delayed the discovery of the Carlin trend gold deposits. Once thought to be barren, Paleozoic carbonaceous sedimentary rocks in Nevada were not explored until Newmont Mining geologists re-evaluated models for the introduction of hydrothermal fluids into sedimentary rocks along major fault networks. They applied the new insights in their exploration programs, leading to discovery of gold-rich pyrite.
Incorporating an AI tool into an exploration workflow will lead to step changes in results. Without any preconceptions, AI tools can analyse data and provoke new ways of thinking that will lead to new discoveries.
The how
The use of machine learning to deliver mineral prospectivity models has been an increasingly accepted method for validating exploration potential in a region. Many mineral prospectivity models have been published that look at diverse mineral systems, including unconformity-related uranium (Bruce et al., 2020), magmatic nickel-copper-PGEs (Lawley et al., 2021; Farahbakhsh et al., 2023), orogenic gold (Maepa et al., 2021), sediment-hosted Zn-Pb (Lawley et al., 2022), carbonatite-hosted REE (Parsa et al., 2024), and porphyry copper-gold (Fu et al., 2023), amongst others. What these studies have in common is the application of various statistical methods of machine learning that aim to define prospectivity in areas away from known deposits of the target commodity.
There are typically three approaches to this end: data-driven, knowledge-driven and a hybrid of the two in which data and knowledge-driven approaches are combined (Bonham-Carter, 1995; Jooshaki et al., 2021).
Knowledge-driven approaches take a conceptual model for mineral deposit formation typically using a mineral systems framework, and linking features of the mineral system model to mappable criteria in data. An example is the knowledge-driven mineral prospectivity mapping of iron oxide copper-gold (IOCG) deposits in Australia by Skirrow et al. (2019) which contains a comprehensive description of the mineral system components from the fluid and metal sources, the energy drivers, the crustal architecture and ore depositional gradients. The types of data used in this approach also include products such as geological maps (surface mapping, or interpreted basement geology etc), which contain features that have been derived from expert domain knowledge. As the model is only looking for locations that meet criteria established by the user, this can cause a bias towards what is considered important by the experts rather than seeking out new patterns in the data.
Data-driven prospectivity is solely based on objective data such as geophysics and geochemistry. These data types are numerical. They give the machine learning algorithms a series of numbers in a multi-dimensional framework that can be compared within a spatial context to inform areas of numerical similarity, hence prospectivity. Machine-learning models such as Random Forest, Support Vector Machines, Neural Networks, or clustering methods are commonly used to analyse the data.
In practice, a hybrid approach is often used that takes elements of both systems and blends the results to achieve a combined assessment of mineral potential for an area.
The what

Fleet Space Technologies’ end-to-end mineral exploration solution, ExoSphere, combines satellite connectivity, remote 3D data acquisition, and AI to accelerate the discovery of critical minerals. Known for its proprietary real-time Ambient Noise Tomography (ANT), ExoSphere delivers 3D subsurface imaging with remarkable fidelity up to depths of 4km, or more depending on survey design. Fleet Space’s smart seismic sensors, Geodes, are 3-20x more sensitive than other geophones and enable real-time quality control via satellite connectivity to optimise high quality, remote data collection. The scale invariance of ExoSphere’s real-time 3D imaging enables multi-scale 3D data acquisition, effectively narrowing the search space from regional to local scales. This 3D data is a vital substrate for training AI models - enabling multimodal data integration, 3D insight visualisation and targeting recommendations.
ExoSphere’s end-to-end capabilities combine real-time 3D imaging, multiphysics data integration, and Fleet Space’s proprietary AI platform to deliver custom AI-driven target recommendations and real-time model calibration. This enables exploration data to be synthesized into actionable insights in real-time, identifying highly prospective zones with potential for mineralisation.
Partnering with the machine
Fleet Space is also pioneering a new framework for AI development – explorers-in-the-loop (EITL) – a foundational step for building AI tools that address challenges of onsite exploration teams and enhance their capabilities. EITL is optimised for collaboration between explorers and AI systems, ensuring advanced computational analysis and the nuanced understanding of explorers drive the evolutionary course of AI in mineral discovery.
In EITL, explorers are involved as drivers decision-making stages, such as training, testing, and data interpretation, to drive accuracy and alignment with their exploration objectives, mineral systems knowledge, and project-level objectives. EITL’s collaborative ‘bottom-up’ approach to AI development is fundamental to enhance data-driven decision making in complex and high-stakes scenarios where the judgment of explorers and AI are critical to achieve more efficient exploration outcomes and maximise learning. This creates a continuous feedback loop between explorers and AI systems, ensuring the course of AI development reflects the needs and challenges faced by explorers while enhancing the precision and efficiency of drill targeting at scale.
By leveraging the predictive capabilities of AI with explorers-in-the-loop, ExoSphere reveals hidden geological insights with data-driven machine learning and in-house experts to systematically narrow the exploration search space and maximise ROI with more accurate drill targets.
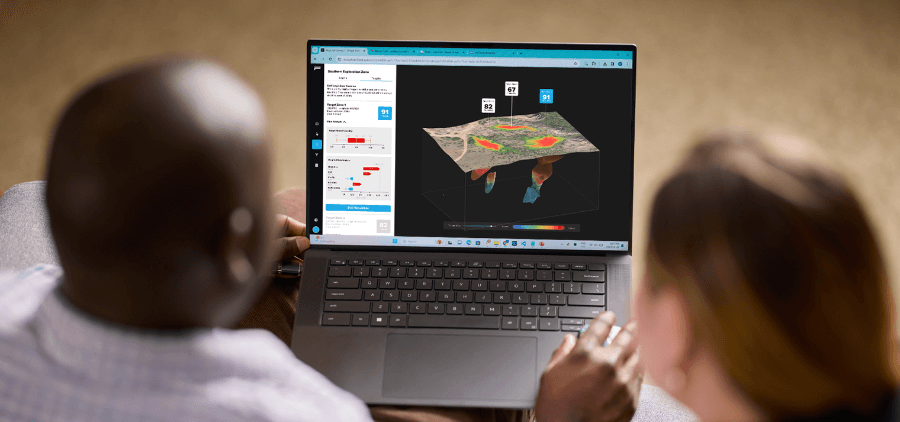
Together, we can solve complex geological challenges
Fleet Space Technologies’ team of geoscience experts is here to help you navigate your unique exploration needs. Contact us today to discuss your needs or request a demo to see how ExoSphere can drive superior exploration outcomes.
References
Bonham-Carter, G., 1995, Geographic information systems for geoscientists: Modelling with GIS: Computer Methods in the Geosciences, Amsterdam, Netherlands, Pergamon, 416 p.
Bruce, M., Kreuzer, O., Wilde, A., Buckingham, A., Butera, K., and Bierlein, F., 2020, Unconformity-type uranium systems: A comparative review and predictive modelling of critical genetic factors: Minerals (Basel, Switzerland), v. 10, no. 9, p. 738.
Farahbakhsh, E., Maughan, J., and Müller, R.D., 2023, Prospectivity modelling of critical mineral deposits using a generative adversarial network with oversampling and positive-unlabelled bagging: Ore geology reviews, no. 105665, p. 105665.
Fu, Y., Cheng, Q., Jing, L., Ye, B., and Fu, H., 2023, Mineral prospectivity mapping of porphyry copper deposits based on remote sensing imagery and geochemical data in the Duolong ore district, Tibet: Remote sensing, v. 15, no. 2, p. 439.
Jooshaki, M., Nad, A., and Michaux, S., 2021, A systematic review on the application of machine learning in exploiting mineralogical data in mining and mineral industry: Minerals (Basel, Switzerland), v. 11, no. 8, p. 816.
Lawley, C.J.M., Tschirhart, V., Smith, J.W., Pehrsson, S.J., Schetselaar, E.M., Schaeffer, A.J., Houlé, M.G., and Eglington, B.M., 2021, Prospectivity modelling of Canadian magmatic Ni (±Cu ± Co ± PGE) sulphide mineral systems: Ore Geology Reviews, v. 132, p. 103985.
Lawley, C.J.M., McCafferty, A.E., Graham, G.E., Huston, D.L., Kelley, K.D., Czarnota, K., Paradis, S., Peter, J.M., Hayward, N., Barlow, M., Emsbo, P., Coyan, J., San Juan, C.A., and Gadd, M.G., 2022, Data–driven prospectivity modelling of sediment–hosted Zn–Pb mineral systems and their critical raw materials: Ore geology reviews, v. 141, no. 104635, p. 104635.
Olivier, G.; Borg, B.; Trevor, L.; Combeau, B.; Dales, P.; Gordon, J.; Chaurasia, H.; Pearson, M. Fleet’s Geode: A Breakthrough Sensor for Real-Time Ambient Seismic Noise Tomography over DtS-IoT. Sensors 2022, 22, 8372.
Maepa, F., Smith, R.S., and Tessema, A., 2021, Support vector machine and artificial neural network modelling of orogenic gold prospectivity mapping in the Swayze greenstone belt, Ontario, Canada: Ore geology reviews, v. 130, no. 103968, p. 103968.
Parsa, M., Lawley, C.J.M., Cumani, R., Schetselaar, E., Harris, J., Lentz, D.R., Zhang, S.E., and Bourdeau, J., 2024, Predictive modeling of Canadian carbonatite-hosted REE +/− Nb deposits: Natural resources research.
Skirrow, R.G., Murr, J., Schofield, A., Huston, D.L., van der Wielen, S., Czarnota, K., Coghlan, R., Highet, L.M., Connolly, D., Doublier, M., and Duan, J., 2019, Mapping iron oxide Cu-Au (IOCG) mineral potential in Australia using a knowledge-driven mineral systems-based approach: Ore Geology Reviews, p. 103011.